Note
Go to the end to download the full example code.
Train, convert and predict with ONNX Runtime#
This example demonstrates an end to end scenario starting with the training of a machine learned model to its use in its converted from.
Train a logistic regression#
The first step consists in retrieving the iris datset.
Then we fit a model.
We compute the prediction on the test set and we show the confusion matrix.
[[11 0 0]
[ 0 10 2]
[ 0 0 15]]
Conversion to ONNX format#
We use module sklearn-onnx to convert the model into ONNX format.
from skl2onnx import convert_sklearn # noqa: E402
from skl2onnx.common.data_types import FloatTensorType # noqa: E402
initial_type = [("float_input", FloatTensorType([None, 4]))]
onx = convert_sklearn(clr, initial_types=initial_type)
with open("logreg_iris.onnx", "wb") as f:
f.write(onx.SerializeToString())
We load the model with ONNX Runtime and look at its input and output.
import onnxruntime as rt # noqa: E402
sess = rt.InferenceSession("logreg_iris.onnx", providers=rt.get_available_providers())
print(f"input name='{sess.get_inputs()[0].name}' and shape={sess.get_inputs()[0].shape}")
print(f"output name='{sess.get_outputs()[0].name}' and shape={sess.get_outputs()[0].shape}")
input name='float_input' and shape=[None, 4]
output name='output_label' and shape=[None]
We compute the predictions.
input_name = sess.get_inputs()[0].name
label_name = sess.get_outputs()[0].name
import numpy # noqa: E402
pred_onx = sess.run([label_name], {input_name: X_test.astype(numpy.float32)})[0]
print(confusion_matrix(pred, pred_onx))
[[11 0 0]
[ 0 10 0]
[ 0 0 17]]
The prediction are perfectly identical.
Probabilities#
Probabilities are needed to compute other relevant metrics such as the ROC Curve. Let’s see how to get them first with scikit-learn.
prob_sklearn = clr.predict_proba(X_test)
print(prob_sklearn[:3])
[[9.49737096e-03 9.18002566e-01 7.25000634e-02]
[9.33228648e-01 6.67709138e-02 4.38521241e-07]
[9.74890047e-01 2.51097982e-02 1.55069701e-07]]
And then with ONNX Runtime. The probabilies appear to be
prob_name = sess.get_outputs()[1].name
prob_rt = sess.run([prob_name], {input_name: X_test.astype(numpy.float32)})[0]
import pprint # noqa: E402
pprint.pprint(prob_rt[0:3])
[{0: 0.009497367776930332, 1: 0.9180026054382324, 2: 0.0725000649690628},
{0: 0.9332286715507507, 1: 0.06677091121673584, 2: 4.385210274904239e-07},
{0: 0.9748900532722473, 1: 0.025109782814979553, 2: 1.5506961403843889e-07}]
Let’s benchmark.
from timeit import Timer # noqa: E402
def speed(inst, number=5, repeat=10):
timer = Timer(inst, globals=globals())
raw = numpy.array(timer.repeat(repeat, number=number))
ave = raw.sum() / len(raw) / number
mi, ma = raw.min() / number, raw.max() / number
print(f"Average {ave:1.3g} min={mi:1.3g} max={ma:1.3g}")
return ave
print("Execution time for clr.predict")
speed("clr.predict(X_test)")
print("Execution time for ONNX Runtime")
speed("sess.run([label_name], {input_name: X_test.astype(numpy.float32)})[0]")
Execution time for clr.predict
Average 7.35e-05 min=6.6e-05 max=0.000113
Execution time for ONNX Runtime
Average 1.84e-05 min=1.71e-05 max=2.48e-05
1.8441459999394283e-05
Let’s benchmark a scenario similar to what a webservice experiences: the model has to do one prediction at a time as opposed to a batch of prediction.
def loop(X_test, fct, n=None):
nrow = X_test.shape[0]
if n is None:
n = nrow
for i in range(n):
im = i % nrow
fct(X_test[im : im + 1])
print("Execution time for clr.predict")
speed("loop(X_test, clr.predict, 50)")
def sess_predict(x):
return sess.run([label_name], {input_name: x.astype(numpy.float32)})[0]
print("Execution time for sess_predict")
speed("loop(X_test, sess_predict, 50)")
Execution time for clr.predict
Average 0.00264 min=0.00234 max=0.0034
Execution time for sess_predict
Average 0.000311 min=0.000306 max=0.00034
0.0003108499999984815
Let’s do the same for the probabilities.
print("Execution time for predict_proba")
speed("loop(X_test, clr.predict_proba, 50)")
def sess_predict_proba(x):
return sess.run([prob_name], {input_name: x.astype(numpy.float32)})[0]
print("Execution time for sess_predict_proba")
speed("loop(X_test, sess_predict_proba, 50)")
Execution time for predict_proba
Average 0.00329 min=0.00326 max=0.0034
Execution time for sess_predict_proba
Average 0.000312 min=0.000307 max=0.000335
0.00031179584000028625
This second comparison is better as ONNX Runtime, in this experience, computes the label and the probabilities in every case.
Benchmark with RandomForest#
We first train and save a model in ONNX format.
from sklearn.ensemble import RandomForestClassifier # noqa: E402
rf = RandomForestClassifier(n_estimators=10)
rf.fit(X_train, y_train)
initial_type = [("float_input", FloatTensorType([1, 4]))]
onx = convert_sklearn(rf, initial_types=initial_type)
with open("rf_iris.onnx", "wb") as f:
f.write(onx.SerializeToString())
We compare.
sess = rt.InferenceSession("rf_iris.onnx", providers=rt.get_available_providers())
def sess_predict_proba_rf(x):
return sess.run([prob_name], {input_name: x.astype(numpy.float32)})[0]
print("Execution time for predict_proba")
speed("loop(X_test, rf.predict_proba, 50)")
print("Execution time for sess_predict_proba")
speed("loop(X_test, sess_predict_proba_rf, 50)")
Execution time for predict_proba
Average 0.0219 min=0.0216 max=0.0231
Execution time for sess_predict_proba
Average 0.000305 min=0.0003 max=0.000331
0.0003054976200019155
Let’s see with different number of trees.
measures = []
for n_trees in range(5, 51, 5):
print(n_trees)
rf = RandomForestClassifier(n_estimators=n_trees)
rf.fit(X_train, y_train)
initial_type = [("float_input", FloatTensorType([1, 4]))]
onx = convert_sklearn(rf, initial_types=initial_type)
with open(f"rf_iris_{n_trees}.onnx", "wb") as f:
f.write(onx.SerializeToString())
sess = rt.InferenceSession(f"rf_iris_{n_trees}.onnx", providers=rt.get_available_providers())
def sess_predict_proba_loop(x):
return sess.run([prob_name], {input_name: x.astype(numpy.float32)})[0] # noqa: B023
tsk = speed("loop(X_test, rf.predict_proba, 25)", number=5, repeat=4)
trt = speed("loop(X_test, sess_predict_proba_loop, 25)", number=5, repeat=4)
measures.append({"n_trees": n_trees, "sklearn": tsk, "rt": trt})
from pandas import DataFrame # noqa: E402
df = DataFrame(measures)
ax = df.plot(x="n_trees", y="sklearn", label="scikit-learn", c="blue", logy=True)
df.plot(x="n_trees", y="rt", label="onnxruntime", ax=ax, c="green", logy=True)
ax.set_xlabel("Number of trees")
ax.set_ylabel("Prediction time (s)")
ax.set_title("Speed comparison between scikit-learn and ONNX Runtime\nFor a random forest on Iris dataset")
ax.legend()
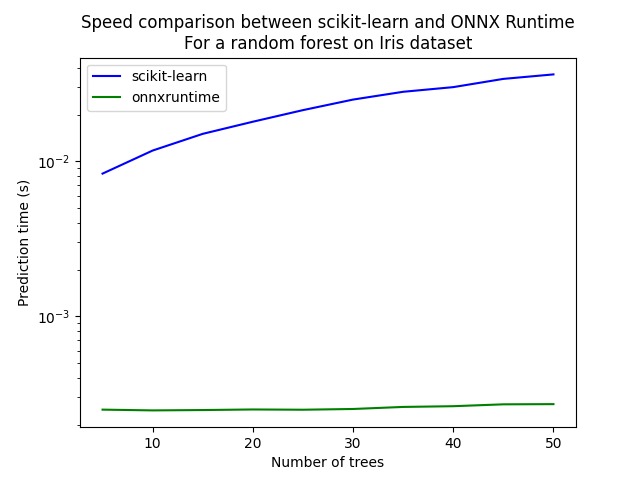
5
Average 0.00803 min=0.00765 max=0.00907
Average 0.000155 min=0.000147 max=0.000178
10
Average 0.0112 min=0.0108 max=0.0122
Average 0.000158 min=0.000149 max=0.00018
15
Average 0.0145 min=0.014 max=0.0154
Average 0.000162 min=0.000154 max=0.000183
20
Average 0.0173 min=0.0169 max=0.0184
Average 0.000161 min=0.000152 max=0.000183
25
Average 0.0205 min=0.0201 max=0.0214
Average 0.000164 min=0.000156 max=0.000186
30
Average 0.0237 min=0.0233 max=0.0247
Average 0.000165 min=0.000157 max=0.000185
35
Average 0.0268 min=0.0262 max=0.0282
Average 0.000166 min=0.000158 max=0.000187
40
Average 0.0295 min=0.0291 max=0.0306
Average 0.000167 min=0.000159 max=0.000189
45
Average 0.0327 min=0.0323 max=0.0338
Average 0.000169 min=0.000162 max=0.000191
50
Average 0.0356 min=0.0353 max=0.0364
Average 0.000171 min=0.000164 max=0.000192
<matplotlib.legend.Legend object at 0x71f100667fa0>
Total running time of the script: (0 minutes 6.383 seconds)